By
Abhiram Sudhir
09.02.2025
12 mins
How Can We Design AI Systems That Are Transparent and Ethically Responsible?
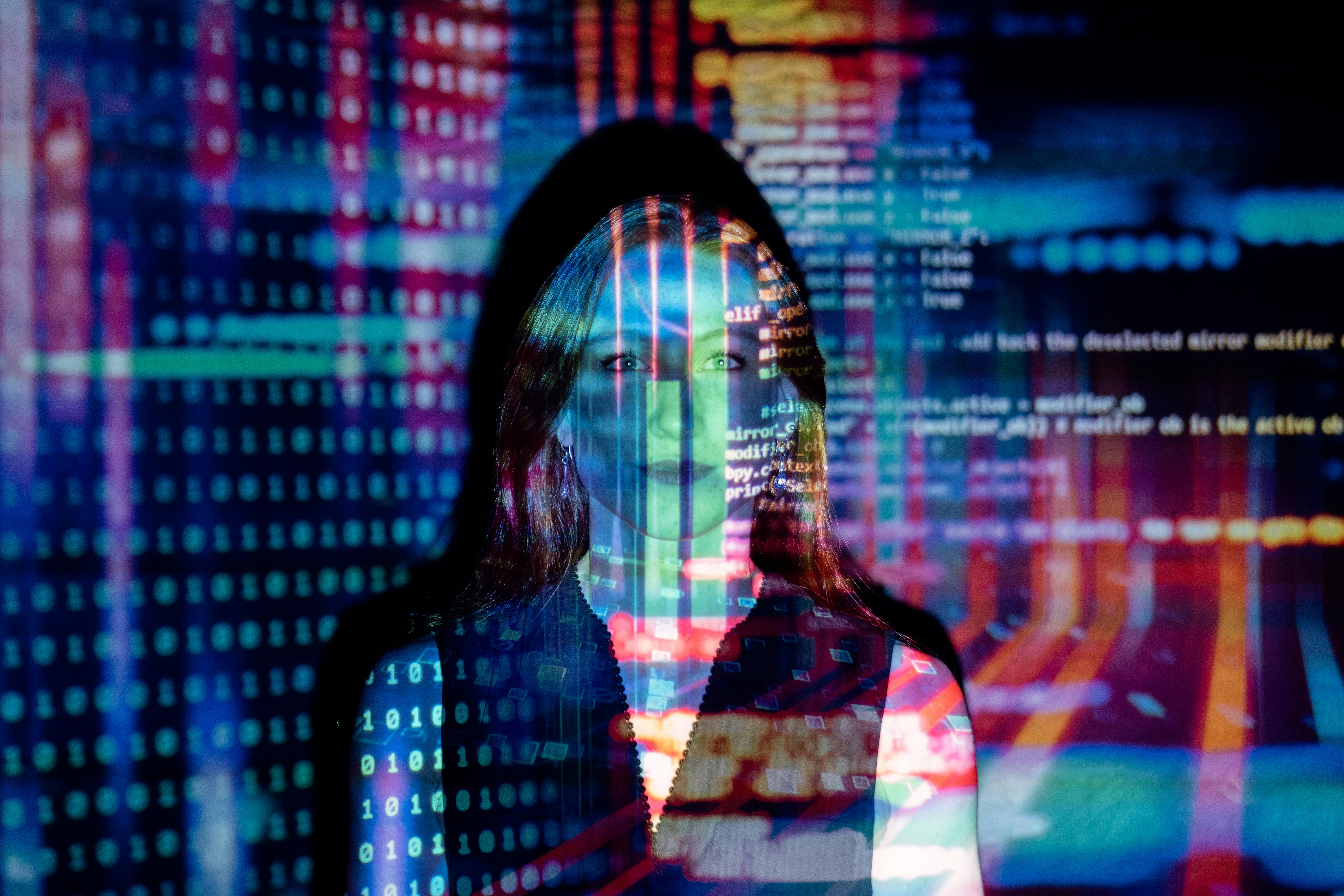
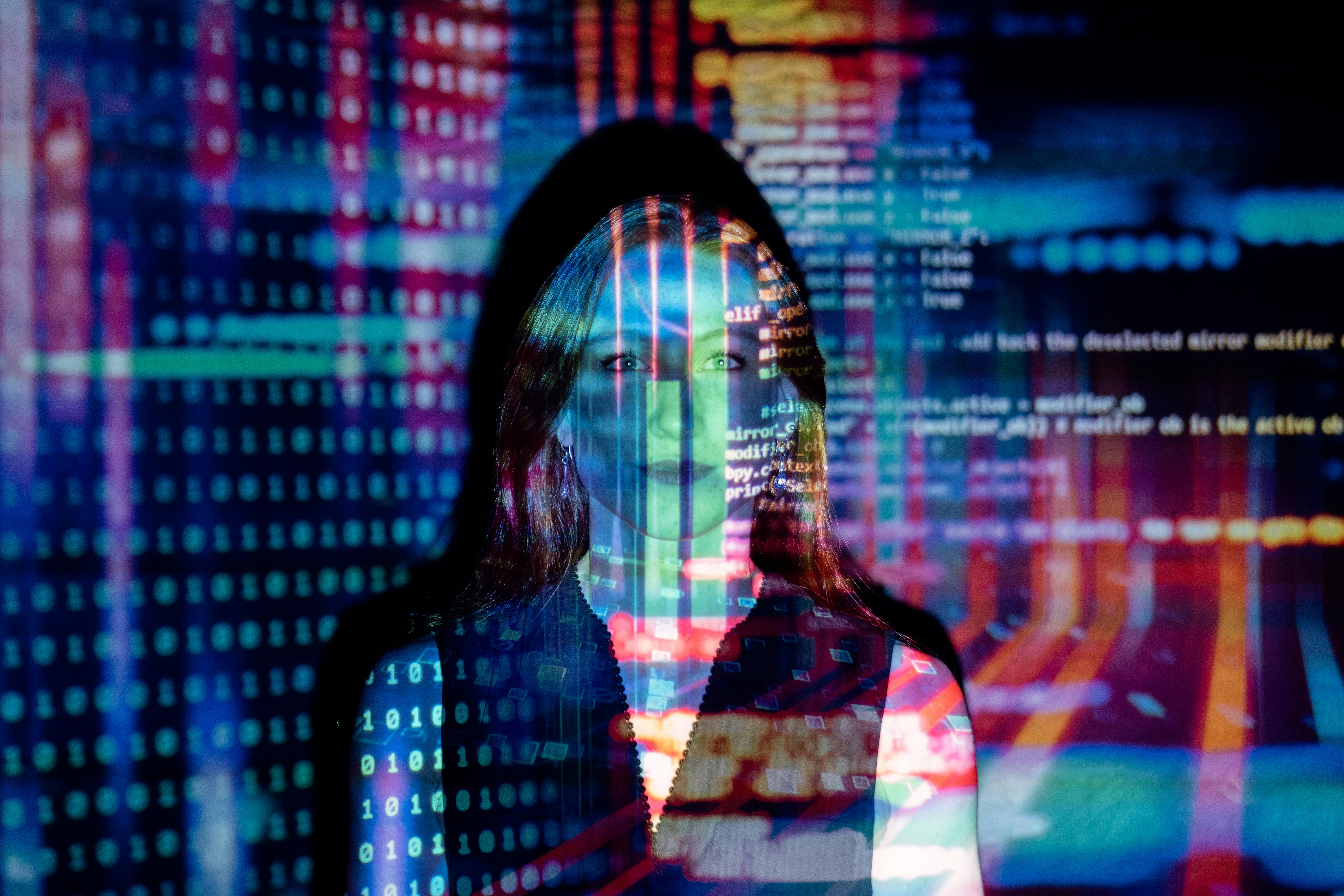
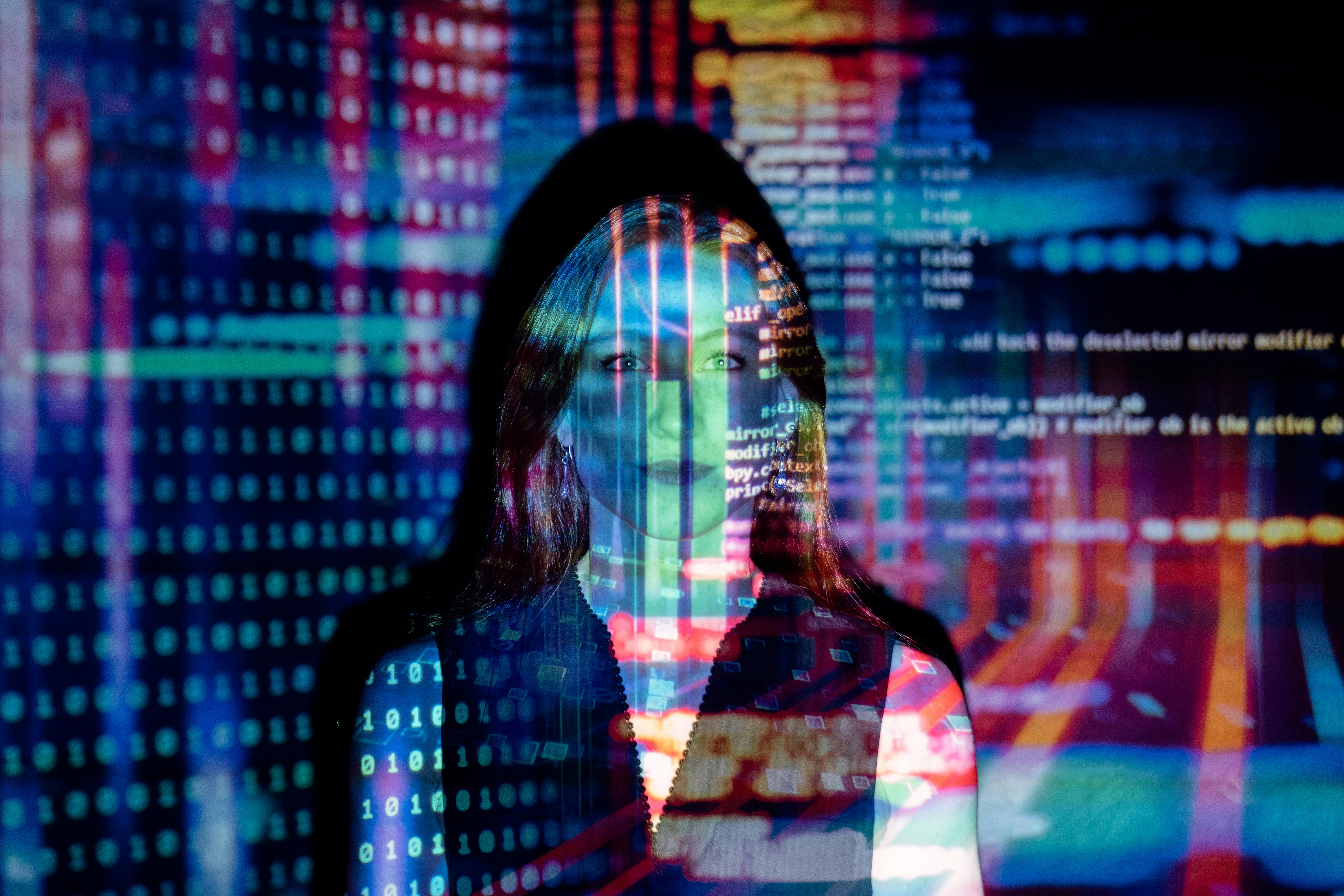
Understanding Ethical AI
Ethical AI refers to the principled development and deployment of artificial intelligence systems that uphold human dignity, rights, and values. It is an evolving domain that reflects the increasing role of AI in shaping our societies, influencing both personal and institutional decisions. In practice, ethical AI requires a multidimensional approach, considering not only the technical functionality of AI systems but also the cultural, social, and political contexts in which these systems operate. Central to ethical AI is the notion of beneficence, that AI should be designed to do good and avoid harm. This includes protecting individual autonomy, ensuring equitable treatment across all social groups, and supporting the common good. The concept also integrates ideas of non-maleficence, justice, and explicability, which means AI systems should be understandable and subject to scrutiny.
For example, in healthcare, an AI system designed to diagnose disease must not only be accurate but must also avoid perpetuating biases that arise from training on data that underrepresents minority populations. Ethical AI therefore encompasses technical accuracy and moral considerations, ensuring that as these systems become more autonomous, they remain aligned with societal values and public trust.
Furthermore, ethical AI goes beyond simply following rules or guidelines, it necessitates embedding moral reasoning into system design and organizational culture. This includes anticipating long-term impacts, creating mechanisms for ethical reflection, and involving ethicists, legal experts, and affected communities throughout the AI lifecycle.
Human-Centered Values and Wellbeing
Designing AI systems around human centered values means creating technologies that empower individuals and communities, rather than displacing or marginalizing them. At its core, this principle acknowledges the fundamental dignity of every human being, advocating for the design of AI that enhances human potential and promotes societal wellbeing. This commitment begins at the level of intent: Why is the AI system being created? Whose problems is it solving? And who might be harmed by its deployment? Human centered AI systems are designed not just for functionality but for social value. They take into account the emotional, cognitive, and physical needs of users, promoting inclusivity and accessibility for people with diverse abilities, backgrounds, and circumstances.
For instance, an AI platform used in education must not only support learning outcomes but also respect students' privacy, allow for different learning styles, and avoid reinforcing existing inequalities. Similarly, a voice assistant should be usable by people with varying speech patterns, dialects, or disabilities. Human centered AI also emphasizes the need for co design involving users in the creation process. This collaborative approach ensures that technologies are shaped by those who will be most affected by them, increasing their relevance, adoption, and fairness. Moreover, when we embed principles of empathy, cultural awareness, and compassion into the design process, we move from designing for people to designing with people.
Crucially, wellbeing extends beyond individual users to encompass environmental sustainability and the health of entire communities. AI systems that consume significant computational resources, for example, must be evaluated for their carbon footprints, pushing developers to find more sustainable architectures and practices. In this way, ethical AI serves both present and future generations.
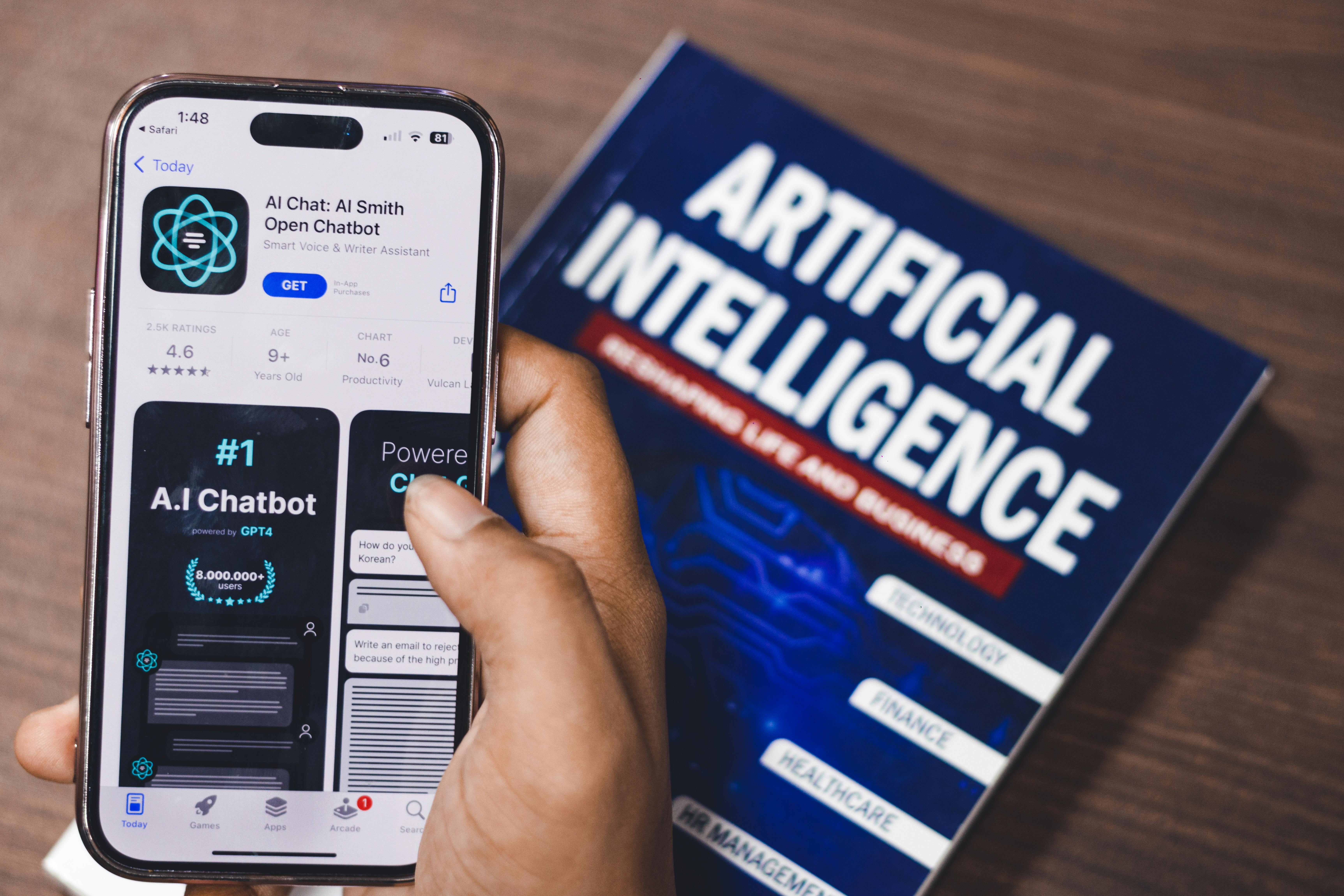
Fairness and Inclusivity in AI
Fairness in AI ensures that systems operate without bias or discrimination, especially against historically marginalized groups. The pursuit of fairness is both a technical and ethical challenge. Technically, developers must address issues such as biased datasets, unfair model assumptions, and unequal outcomes. Ethically, it requires engaging with questions of justice, representation, and human dignity. Bias in AI systems often originates from the data they are trained on. If the training data reflects existing social inequalities, these can be encoded and amplified in AI predictions. For instance, a facial recognition system trained predominantly on lighter skinned individuals may have significantly lower accuracy for darker-skinned faces. This leads not only to unequal service quality but also to serious risks, such as wrongful identification in law enforcement contexts.
Ensuring fairness means applying fairness metrics, statistical techniques to detect disparities in AI outputs across different demographic groups. These include demographic parity, equal opportunity, and individual fairness, each addressing fairness from different angles. But fairness also demands contextual awareness, understanding the specific social and historical dynamics of the domain in which the AI operates.
Inclusion, on the other hand, relates to the participation of diverse voices in AI development and governance. This includes gender diversity, racial representation, neurodiversity, and socio-economic diversity among development teams. Inclusive design ensures that AI systems are sensitive to the needs of all users, not just the dominant group. It also helps uncover hidden assumptions that may otherwise go unchallenged. A best practice in this regard is conducting algorithmic impact assessments, systematic evaluations of how an AI system might affect different stakeholders. This process, which mirrors environmental impact assessments, includes consultations with community groups, subject matter experts, and ethical review boards.
Privacy, Security, and Data Governance
AI systems thrive on data, but with data comes the critical responsibility of privacy protection and ethical data governance. In our increasingly digitized world, where data is collected through everything from smart devices to social media, ensuring privacy is a foundational ethical imperative.
Privacy is not merely a legal issue, it is a human right. AI systems must be designed to protect user autonomy, preserve anonymity when required, and prevent data misuse. This involves implementing strong data minimization policies (only collecting what is necessary), secure storage, and user control over how data is shared or used.
Security complements privacy. It refers to protecting AI systems from unauthorized access, adversarial attacks, and breaches that could compromise sensitive data or system behavior. A healthcare AI misdiagnosing a patient due to a cyberattack is not just a technical failure, it’s an ethical catastrophe. Data governance structures are needed to manage how data is sourced, labeled, stored, and accessed. Responsible data governance includes setting standards for transparency (e.g., data lineage and provenance), accountability (e.g., who is responsible for data breaches), and fairness (e.g., removing systemic bias from datasets).
One increasingly adopted framework is differential privacy, which enables AI systems to analyze data trends without exposing individual records. Another is federated learning, where models are trained across decentralized devices without transferring raw data to a central server, thereby preserving privacy. In high stakes domains, such as finance or justice, data used in AI systems must be auditable. Organizations should maintain documentation (often called “model cards” and “data sheets”) that describe the origin, structure, and limitations of the data and models they use. This transparency helps identify risks and improves public confidence in AI systems.
Transparency and Explainability
Transparency and explainability are essential for ensuring that AI systems are understandable and trustworthy. In many AI applications, especially those using deep learning, decisions are made through processes that are not easily interpretable by humans. This opacity creates what’s known as a "black box," where the inner workings of the system are hidden from users, developers, and regulators. Explainability means providing clear, meaningful information about how AI decisions are made. This is particularly important in high stakes situations, such as healthcare diagnoses, loan approvals, or parole decisions. When people are denied services or opportunities by an algorithm, they deserve an explanation they can comprehend and act upon.
Methods for achieving explainability include:
· Feature importance analysis, which shows which data inputs were most influential in a decision.
· Local Interpretable Model-agnostic Explanations (LIME), which approximates complex models with simpler ones for specific predictions.
· Counterfactual explanations, which answer the question: “What would have changed the outcome?”
· Model visualizations, including decision trees, heatmaps, and other graphical tools to demystify AI operations.
Beyond the technical layer, transparency also includes disclosure: Users should know when they are interacting with an AI system and how their data is being used. Organizations should also provide information on their AI models' capabilities, limitations, and potential risks.
From a governance perspective, transparency is a prerequisite for auditability. Regulators and oversight bodies need access to documentation and model behavior to evaluate compliance with legal and ethical standards. Increasingly, companies are being asked to publish transparency reports, detailing how their AI systems function and what steps they’ve taken to ensure fairness and accountability. Transparency builds public trust. In a world where misinformation and opaque technologies proliferate, offering clarity is not only ethical, it’s strategic.
Accountability and Contestability
Accountability ensures that someone can be held responsible when AI systems cause harm. In complex systems with many actors, from developers and data scientists to executives and policymakers, it’s easy for responsibility to become diluted. Ethical AI requires establishing clear lines of responsibility across the AI lifecycle.
There are several forms of accountability:
· Legal accountability, where laws define liability for harm caused by AI systems.
· Moral accountability, where organizations take responsibility for the societal impact of their technologies.
· Organizational accountability, where specific roles (e.g., AI ethics officer) are assigned to oversee responsible development.
Contestability means giving users the power to challenge or appeal AI decisions. This is especially important when AI systems make consequential decisions that affect people’s lives, such as eligibility for government benefits, job hiring, or immigration status.
To enable contestability, systems must:
· Keep audit trails records of how decisions were made.
· Offer appeals processes that involve human oversight.
· Provide access to inputs and reasoning used in the AI decision.
· Ensure timely resolution and responsiveness to challenges.
Building contestability into AI systems also improves their robustness. When feedback loops exist, organizations can learn from errors and refine their models to prevent recurrence. Without accountability and contestability, AI systems risk becoming instruments of unassailable authority, eroding democratic values and human rights. Ethical AI restores balance by ensuring systems remain subordinate to human judgment.
Regulatory and Ethical Frameworks
Effective governance of AI requires a combination of regulatory frameworks, ethical guidelines, and industry standards. Globally, governments and organizations are racing to develop policies that balance innovation with responsibility.
Some prominent frameworks include:
· Australia’s AI Ethics Principles, which offer voluntary guidelines for organizations to promote responsible AI development.
· EU’s Artificial Intelligence Act, which categorizes AI systems by risk level and applies proportionate regulatory requirements.
· OECD AI Principles, which promote inclusive growth, human-centered values, transparency, robustness, and accountability.
· IEEE Ethically Aligned Design, which provides recommendations for aligning AI with ethical principles across sectors.
These frameworks vary in scope and enforcement but share a common goal: to ensure that AI systems are aligned with fundamental rights and democratic values. Many of them emphasize the need for risk-based regulation, where the level of oversight depends on the potential harm of the AI application.
However, regulation alone is not enough. Ethical frameworks provide the moral compass that guides organizations beyond what’s legally required. These frameworks encourage self assessment, peer accountability, and public transparency. Leading companies are creating internal ethics boards, publishing AI principles, and training employees in ethical decision making. Ultimately, regulation and ethics must work hand in hand. Ethical principles guide behavior, while regulation enforces boundaries. Together, they create an ecosystem where responsible innovation can thrive.
Building a Culture of Responsible Innovation
Creating transparent and ethically responsible AI is not just a technical challenge, it’s a cultural transformation. Organizations must cultivate values that prioritize ethics, social impact, and human dignity alongside profitability and performance.
A culture of responsible innovation is one where ethical reflection is embedded into every stage of the AI lifecycle, from problem definition to design, deployment, and monitoring. This requires leadership commitment, interdisciplinary collaboration, and continuous education.
Steps to building this culture include:
· Establishing ethics boards or review committees that guide AI development.
· Hiring ethicists, social scientists, and community representatives into AI teams.
· Training staff on responsible AI principles and real world case studies.
· Incorporating ethical design patterns, such as user consent flows, fail safes, and equitable defaults.
· Providing feedback loops, where users can report issues or offer suggestions.
This cultural shift also means embracing humility and learning from failure. Organizations must be willing to revise or even withdraw AI systems that fail to meet ethical standards. Transparency in admitting mistakes and sharing lessons learned can enhance trust and inspire industry-wide improvements.
Finally, responsible innovation is about purpose. Why build this system? Whose life will it improve? By keeping humanity at the heart of technological progress, we ensure that AI serves as a force for good amplifying our values, supporting our communities, and creating a future that is not only intelligent but wise.
Your Next Step Starts Here
Got a bold idea or a tricky problem? We’re here to help. We work with individuals, startups, and businesses to design solutions that matter. Let’s team up and build something great together.
Your Next Step Starts Here
Got a bold idea or a tricky problem? We’re here to help. We work with individuals, startups, and businesses to design solutions that matter. Let’s team up and build something great together.
Your Next Step Starts Here
Got a bold idea or a tricky problem? We’re here to help. We work with individuals, startups, and businesses to design solutions that matter. Let’s team up and build something great together.
By
Abhiram Sudhir
09.02.2025
12 mins
How Can We Design AI Systems That Are Transparent and Ethically Responsible?
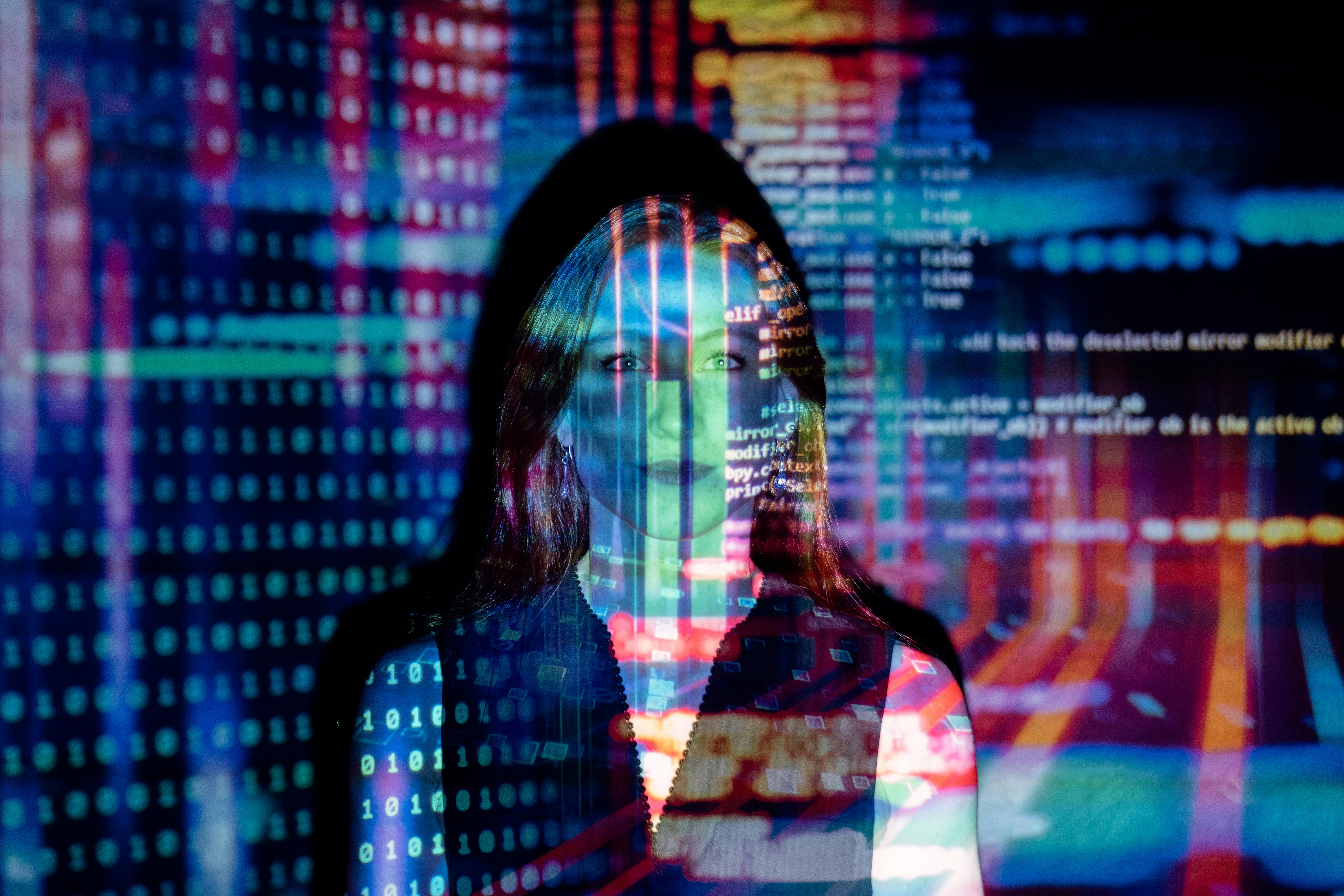
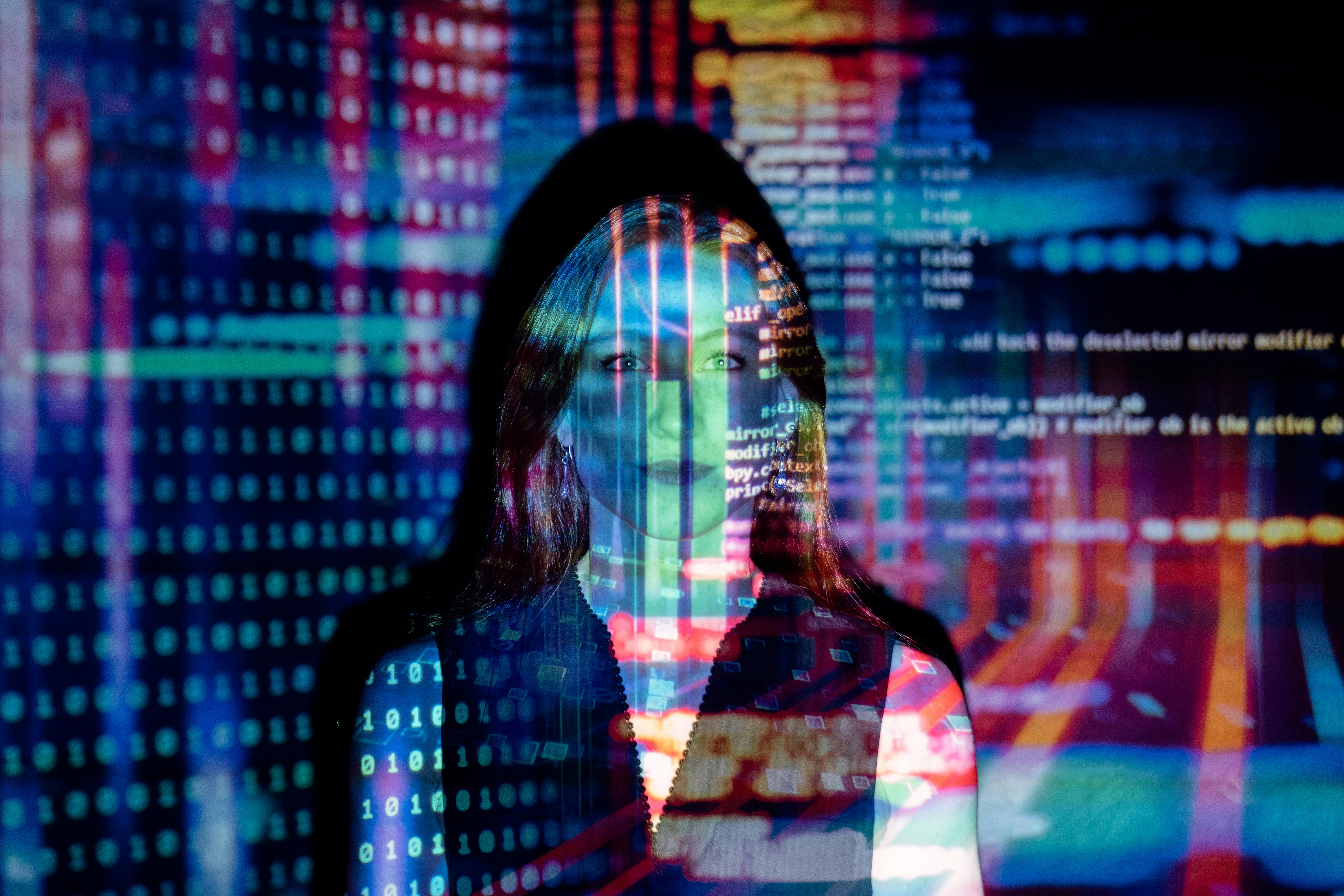
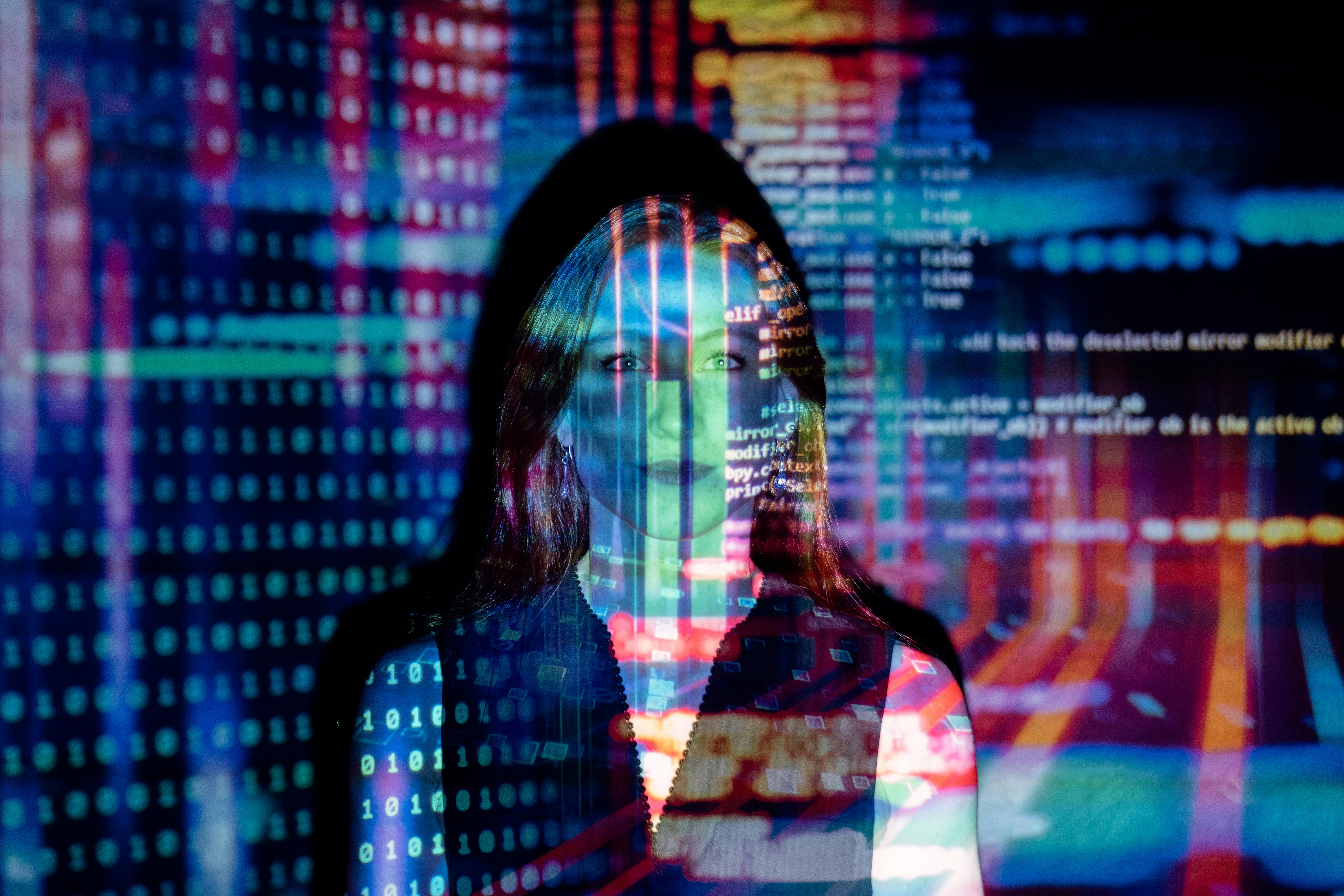
Understanding Ethical AI
Ethical AI refers to the principled development and deployment of artificial intelligence systems that uphold human dignity, rights, and values. It is an evolving domain that reflects the increasing role of AI in shaping our societies, influencing both personal and institutional decisions. In practice, ethical AI requires a multidimensional approach, considering not only the technical functionality of AI systems but also the cultural, social, and political contexts in which these systems operate. Central to ethical AI is the notion of beneficence, that AI should be designed to do good and avoid harm. This includes protecting individual autonomy, ensuring equitable treatment across all social groups, and supporting the common good. The concept also integrates ideas of non-maleficence, justice, and explicability, which means AI systems should be understandable and subject to scrutiny.
For example, in healthcare, an AI system designed to diagnose disease must not only be accurate but must also avoid perpetuating biases that arise from training on data that underrepresents minority populations. Ethical AI therefore encompasses technical accuracy and moral considerations, ensuring that as these systems become more autonomous, they remain aligned with societal values and public trust.
Furthermore, ethical AI goes beyond simply following rules or guidelines, it necessitates embedding moral reasoning into system design and organizational culture. This includes anticipating long-term impacts, creating mechanisms for ethical reflection, and involving ethicists, legal experts, and affected communities throughout the AI lifecycle.
Human-Centered Values and Wellbeing
Designing AI systems around human centered values means creating technologies that empower individuals and communities, rather than displacing or marginalizing them. At its core, this principle acknowledges the fundamental dignity of every human being, advocating for the design of AI that enhances human potential and promotes societal wellbeing. This commitment begins at the level of intent: Why is the AI system being created? Whose problems is it solving? And who might be harmed by its deployment? Human centered AI systems are designed not just for functionality but for social value. They take into account the emotional, cognitive, and physical needs of users, promoting inclusivity and accessibility for people with diverse abilities, backgrounds, and circumstances.
For instance, an AI platform used in education must not only support learning outcomes but also respect students' privacy, allow for different learning styles, and avoid reinforcing existing inequalities. Similarly, a voice assistant should be usable by people with varying speech patterns, dialects, or disabilities. Human centered AI also emphasizes the need for co design involving users in the creation process. This collaborative approach ensures that technologies are shaped by those who will be most affected by them, increasing their relevance, adoption, and fairness. Moreover, when we embed principles of empathy, cultural awareness, and compassion into the design process, we move from designing for people to designing with people.
Crucially, wellbeing extends beyond individual users to encompass environmental sustainability and the health of entire communities. AI systems that consume significant computational resources, for example, must be evaluated for their carbon footprints, pushing developers to find more sustainable architectures and practices. In this way, ethical AI serves both present and future generations.
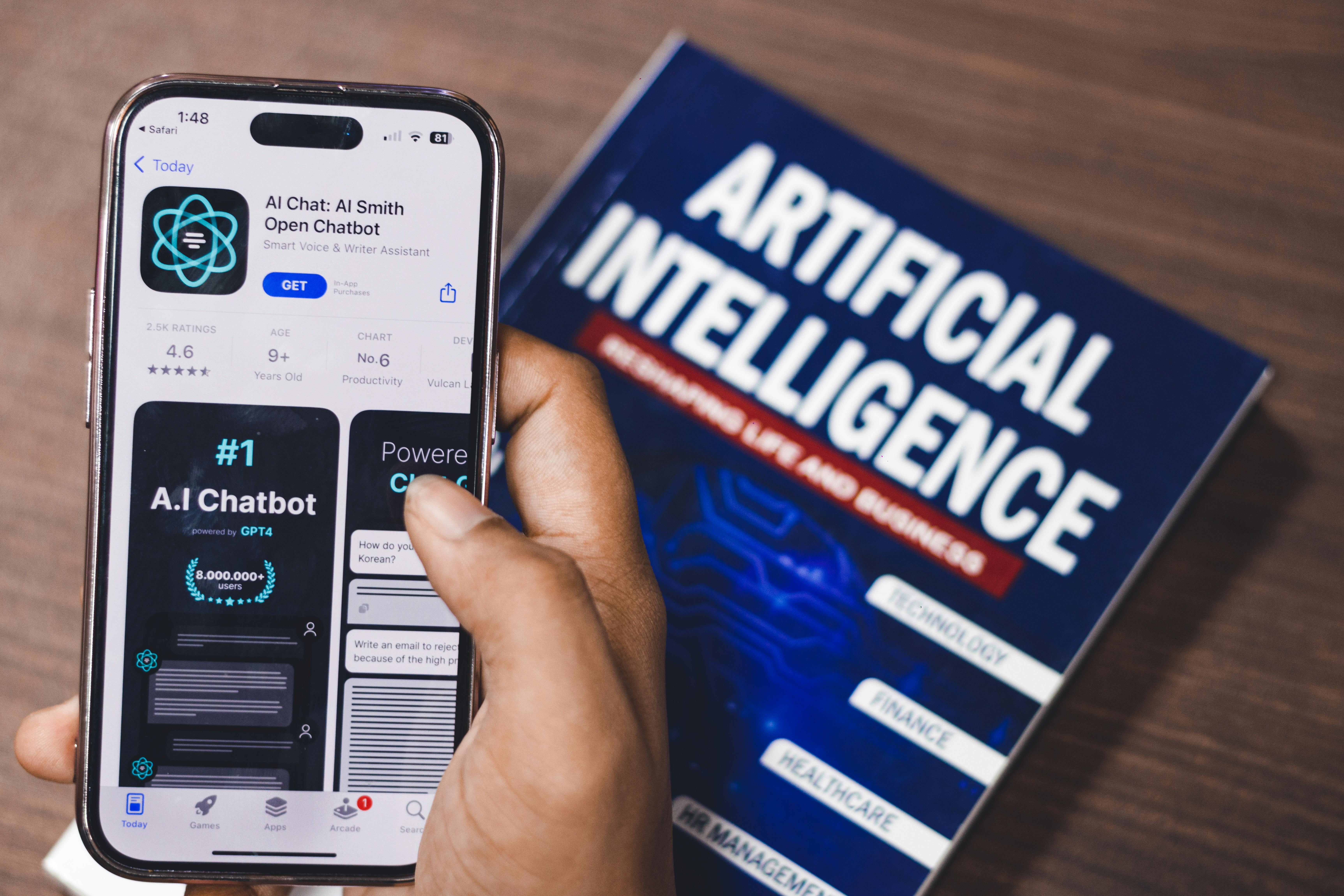
Fairness and Inclusivity in AI
Fairness in AI ensures that systems operate without bias or discrimination, especially against historically marginalized groups. The pursuit of fairness is both a technical and ethical challenge. Technically, developers must address issues such as biased datasets, unfair model assumptions, and unequal outcomes. Ethically, it requires engaging with questions of justice, representation, and human dignity. Bias in AI systems often originates from the data they are trained on. If the training data reflects existing social inequalities, these can be encoded and amplified in AI predictions. For instance, a facial recognition system trained predominantly on lighter skinned individuals may have significantly lower accuracy for darker-skinned faces. This leads not only to unequal service quality but also to serious risks, such as wrongful identification in law enforcement contexts.
Ensuring fairness means applying fairness metrics, statistical techniques to detect disparities in AI outputs across different demographic groups. These include demographic parity, equal opportunity, and individual fairness, each addressing fairness from different angles. But fairness also demands contextual awareness, understanding the specific social and historical dynamics of the domain in which the AI operates.
Inclusion, on the other hand, relates to the participation of diverse voices in AI development and governance. This includes gender diversity, racial representation, neurodiversity, and socio-economic diversity among development teams. Inclusive design ensures that AI systems are sensitive to the needs of all users, not just the dominant group. It also helps uncover hidden assumptions that may otherwise go unchallenged. A best practice in this regard is conducting algorithmic impact assessments, systematic evaluations of how an AI system might affect different stakeholders. This process, which mirrors environmental impact assessments, includes consultations with community groups, subject matter experts, and ethical review boards.
Privacy, Security, and Data Governance
AI systems thrive on data, but with data comes the critical responsibility of privacy protection and ethical data governance. In our increasingly digitized world, where data is collected through everything from smart devices to social media, ensuring privacy is a foundational ethical imperative.
Privacy is not merely a legal issue, it is a human right. AI systems must be designed to protect user autonomy, preserve anonymity when required, and prevent data misuse. This involves implementing strong data minimization policies (only collecting what is necessary), secure storage, and user control over how data is shared or used.
Security complements privacy. It refers to protecting AI systems from unauthorized access, adversarial attacks, and breaches that could compromise sensitive data or system behavior. A healthcare AI misdiagnosing a patient due to a cyberattack is not just a technical failure, it’s an ethical catastrophe. Data governance structures are needed to manage how data is sourced, labeled, stored, and accessed. Responsible data governance includes setting standards for transparency (e.g., data lineage and provenance), accountability (e.g., who is responsible for data breaches), and fairness (e.g., removing systemic bias from datasets).
One increasingly adopted framework is differential privacy, which enables AI systems to analyze data trends without exposing individual records. Another is federated learning, where models are trained across decentralized devices without transferring raw data to a central server, thereby preserving privacy. In high stakes domains, such as finance or justice, data used in AI systems must be auditable. Organizations should maintain documentation (often called “model cards” and “data sheets”) that describe the origin, structure, and limitations of the data and models they use. This transparency helps identify risks and improves public confidence in AI systems.
Transparency and Explainability
Transparency and explainability are essential for ensuring that AI systems are understandable and trustworthy. In many AI applications, especially those using deep learning, decisions are made through processes that are not easily interpretable by humans. This opacity creates what’s known as a "black box," where the inner workings of the system are hidden from users, developers, and regulators. Explainability means providing clear, meaningful information about how AI decisions are made. This is particularly important in high stakes situations, such as healthcare diagnoses, loan approvals, or parole decisions. When people are denied services or opportunities by an algorithm, they deserve an explanation they can comprehend and act upon.
Methods for achieving explainability include:
· Feature importance analysis, which shows which data inputs were most influential in a decision.
· Local Interpretable Model-agnostic Explanations (LIME), which approximates complex models with simpler ones for specific predictions.
· Counterfactual explanations, which answer the question: “What would have changed the outcome?”
· Model visualizations, including decision trees, heatmaps, and other graphical tools to demystify AI operations.
Beyond the technical layer, transparency also includes disclosure: Users should know when they are interacting with an AI system and how their data is being used. Organizations should also provide information on their AI models' capabilities, limitations, and potential risks.
From a governance perspective, transparency is a prerequisite for auditability. Regulators and oversight bodies need access to documentation and model behavior to evaluate compliance with legal and ethical standards. Increasingly, companies are being asked to publish transparency reports, detailing how their AI systems function and what steps they’ve taken to ensure fairness and accountability. Transparency builds public trust. In a world where misinformation and opaque technologies proliferate, offering clarity is not only ethical, it’s strategic.
Accountability and Contestability
Accountability ensures that someone can be held responsible when AI systems cause harm. In complex systems with many actors, from developers and data scientists to executives and policymakers, it’s easy for responsibility to become diluted. Ethical AI requires establishing clear lines of responsibility across the AI lifecycle.
There are several forms of accountability:
· Legal accountability, where laws define liability for harm caused by AI systems.
· Moral accountability, where organizations take responsibility for the societal impact of their technologies.
· Organizational accountability, where specific roles (e.g., AI ethics officer) are assigned to oversee responsible development.
Contestability means giving users the power to challenge or appeal AI decisions. This is especially important when AI systems make consequential decisions that affect people’s lives, such as eligibility for government benefits, job hiring, or immigration status.
To enable contestability, systems must:
· Keep audit trails records of how decisions were made.
· Offer appeals processes that involve human oversight.
· Provide access to inputs and reasoning used in the AI decision.
· Ensure timely resolution and responsiveness to challenges.
Building contestability into AI systems also improves their robustness. When feedback loops exist, organizations can learn from errors and refine their models to prevent recurrence. Without accountability and contestability, AI systems risk becoming instruments of unassailable authority, eroding democratic values and human rights. Ethical AI restores balance by ensuring systems remain subordinate to human judgment.
Regulatory and Ethical Frameworks
Effective governance of AI requires a combination of regulatory frameworks, ethical guidelines, and industry standards. Globally, governments and organizations are racing to develop policies that balance innovation with responsibility.
Some prominent frameworks include:
· Australia’s AI Ethics Principles, which offer voluntary guidelines for organizations to promote responsible AI development.
· EU’s Artificial Intelligence Act, which categorizes AI systems by risk level and applies proportionate regulatory requirements.
· OECD AI Principles, which promote inclusive growth, human-centered values, transparency, robustness, and accountability.
· IEEE Ethically Aligned Design, which provides recommendations for aligning AI with ethical principles across sectors.
These frameworks vary in scope and enforcement but share a common goal: to ensure that AI systems are aligned with fundamental rights and democratic values. Many of them emphasize the need for risk-based regulation, where the level of oversight depends on the potential harm of the AI application.
However, regulation alone is not enough. Ethical frameworks provide the moral compass that guides organizations beyond what’s legally required. These frameworks encourage self assessment, peer accountability, and public transparency. Leading companies are creating internal ethics boards, publishing AI principles, and training employees in ethical decision making. Ultimately, regulation and ethics must work hand in hand. Ethical principles guide behavior, while regulation enforces boundaries. Together, they create an ecosystem where responsible innovation can thrive.
Building a Culture of Responsible Innovation
Creating transparent and ethically responsible AI is not just a technical challenge, it’s a cultural transformation. Organizations must cultivate values that prioritize ethics, social impact, and human dignity alongside profitability and performance.
A culture of responsible innovation is one where ethical reflection is embedded into every stage of the AI lifecycle, from problem definition to design, deployment, and monitoring. This requires leadership commitment, interdisciplinary collaboration, and continuous education.
Steps to building this culture include:
· Establishing ethics boards or review committees that guide AI development.
· Hiring ethicists, social scientists, and community representatives into AI teams.
· Training staff on responsible AI principles and real world case studies.
· Incorporating ethical design patterns, such as user consent flows, fail safes, and equitable defaults.
· Providing feedback loops, where users can report issues or offer suggestions.
This cultural shift also means embracing humility and learning from failure. Organizations must be willing to revise or even withdraw AI systems that fail to meet ethical standards. Transparency in admitting mistakes and sharing lessons learned can enhance trust and inspire industry-wide improvements.
Finally, responsible innovation is about purpose. Why build this system? Whose life will it improve? By keeping humanity at the heart of technological progress, we ensure that AI serves as a force for good amplifying our values, supporting our communities, and creating a future that is not only intelligent but wise.
Your Next Step Starts Here
Got a bold idea or a tricky problem? We’re here to help. We work with individuals, startups, and businesses to design solutions that matter. Let’s team up and build something great together.
Your Next Step Starts Here
Got a bold idea or a tricky problem? We’re here to help. We work with individuals, startups, and businesses to design solutions that matter. Let’s team up and build something great together.
Your Next Step Starts Here
Got a bold idea or a tricky problem? We’re here to help. We work with individuals, startups, and businesses to design solutions that matter. Let’s team up and build something great together.
By
Abhiram Sudhir
09.02.2025
12 mins
How Can We Design AI Systems That Are Transparent and Ethically Responsible?
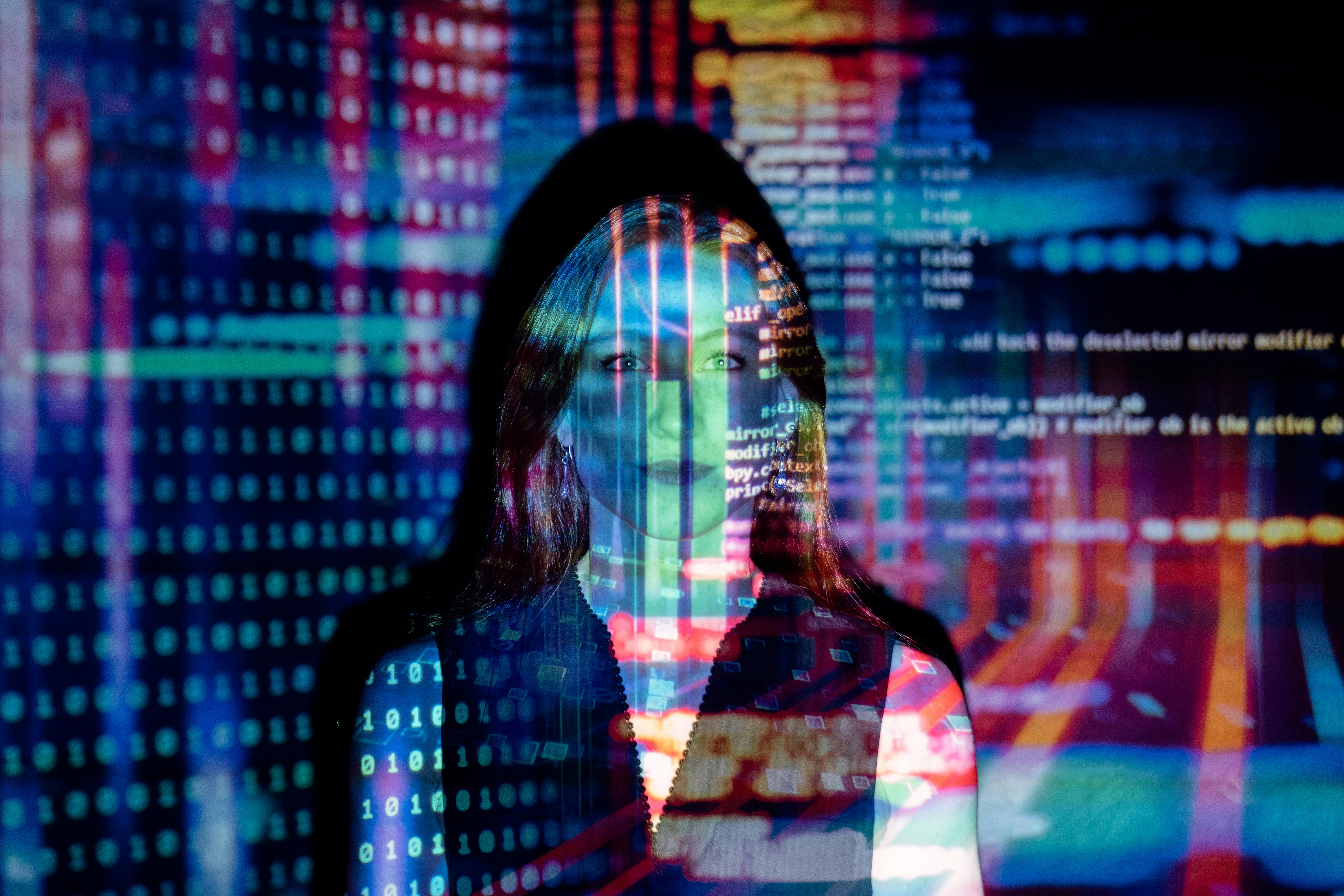
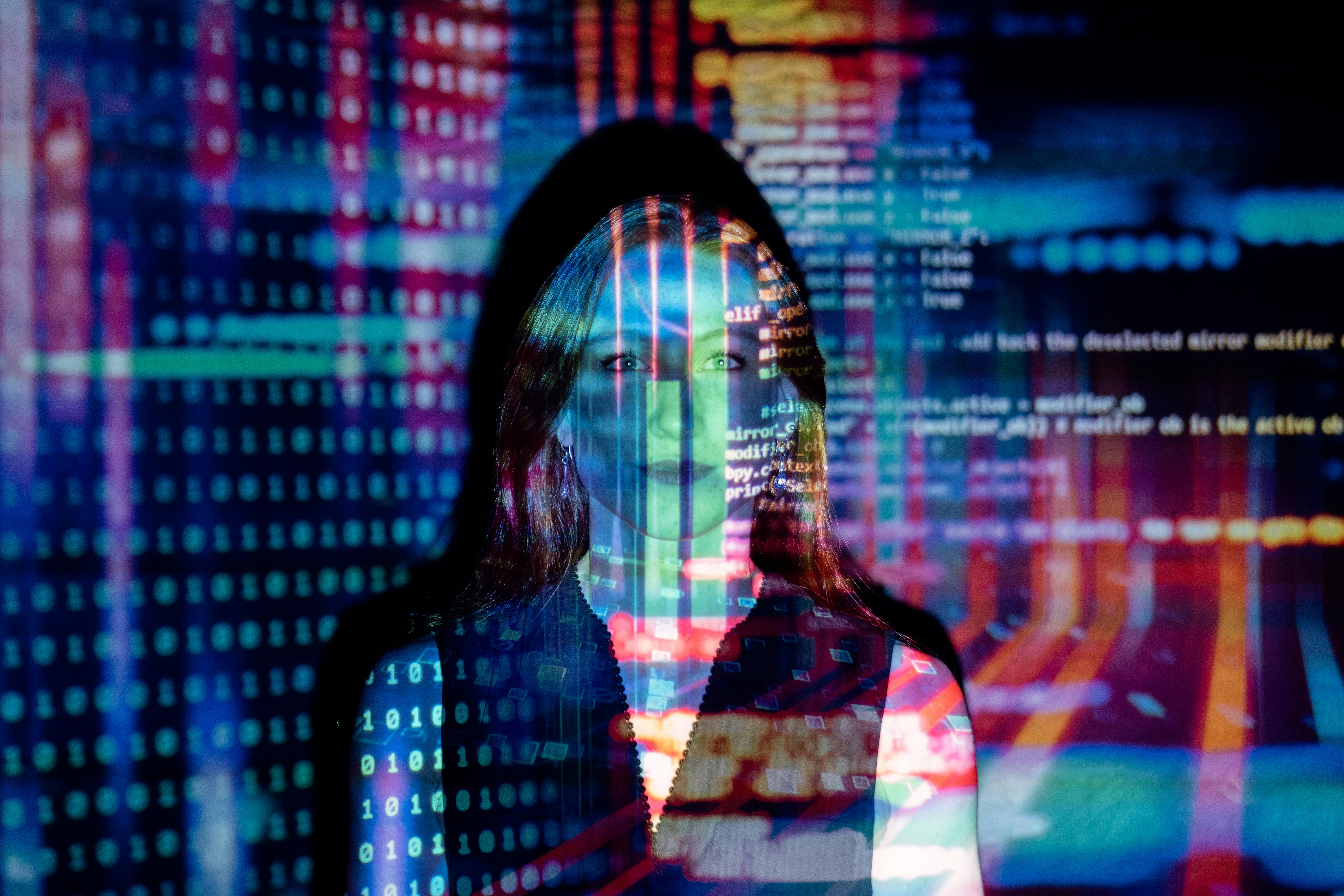
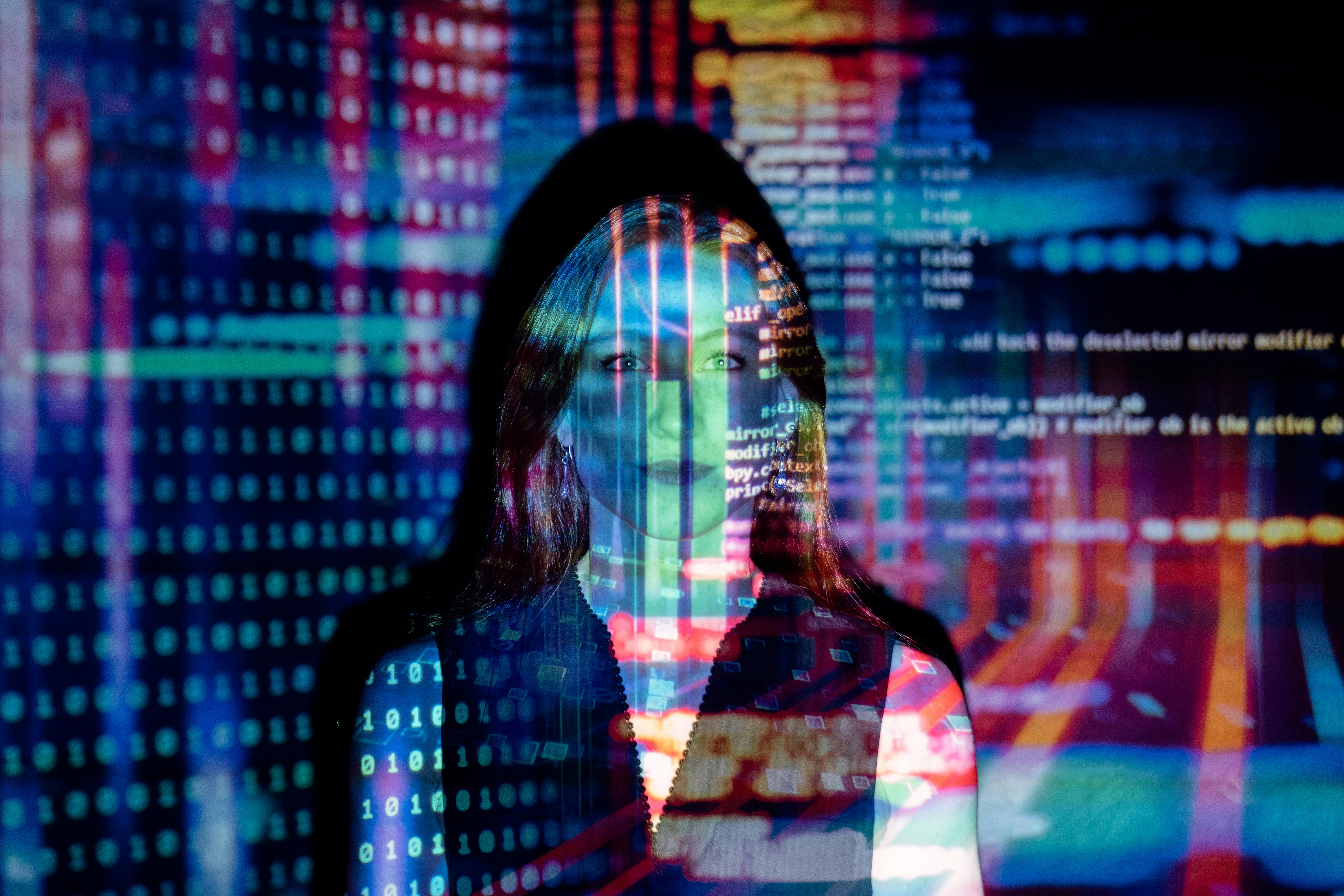
Understanding Ethical AI
Ethical AI refers to the principled development and deployment of artificial intelligence systems that uphold human dignity, rights, and values. It is an evolving domain that reflects the increasing role of AI in shaping our societies, influencing both personal and institutional decisions. In practice, ethical AI requires a multidimensional approach, considering not only the technical functionality of AI systems but also the cultural, social, and political contexts in which these systems operate. Central to ethical AI is the notion of beneficence, that AI should be designed to do good and avoid harm. This includes protecting individual autonomy, ensuring equitable treatment across all social groups, and supporting the common good. The concept also integrates ideas of non-maleficence, justice, and explicability, which means AI systems should be understandable and subject to scrutiny.
For example, in healthcare, an AI system designed to diagnose disease must not only be accurate but must also avoid perpetuating biases that arise from training on data that underrepresents minority populations. Ethical AI therefore encompasses technical accuracy and moral considerations, ensuring that as these systems become more autonomous, they remain aligned with societal values and public trust.
Furthermore, ethical AI goes beyond simply following rules or guidelines, it necessitates embedding moral reasoning into system design and organizational culture. This includes anticipating long-term impacts, creating mechanisms for ethical reflection, and involving ethicists, legal experts, and affected communities throughout the AI lifecycle.
Human-Centered Values and Wellbeing
Designing AI systems around human centered values means creating technologies that empower individuals and communities, rather than displacing or marginalizing them. At its core, this principle acknowledges the fundamental dignity of every human being, advocating for the design of AI that enhances human potential and promotes societal wellbeing. This commitment begins at the level of intent: Why is the AI system being created? Whose problems is it solving? And who might be harmed by its deployment? Human centered AI systems are designed not just for functionality but for social value. They take into account the emotional, cognitive, and physical needs of users, promoting inclusivity and accessibility for people with diverse abilities, backgrounds, and circumstances.
For instance, an AI platform used in education must not only support learning outcomes but also respect students' privacy, allow for different learning styles, and avoid reinforcing existing inequalities. Similarly, a voice assistant should be usable by people with varying speech patterns, dialects, or disabilities. Human centered AI also emphasizes the need for co design involving users in the creation process. This collaborative approach ensures that technologies are shaped by those who will be most affected by them, increasing their relevance, adoption, and fairness. Moreover, when we embed principles of empathy, cultural awareness, and compassion into the design process, we move from designing for people to designing with people.
Crucially, wellbeing extends beyond individual users to encompass environmental sustainability and the health of entire communities. AI systems that consume significant computational resources, for example, must be evaluated for their carbon footprints, pushing developers to find more sustainable architectures and practices. In this way, ethical AI serves both present and future generations.
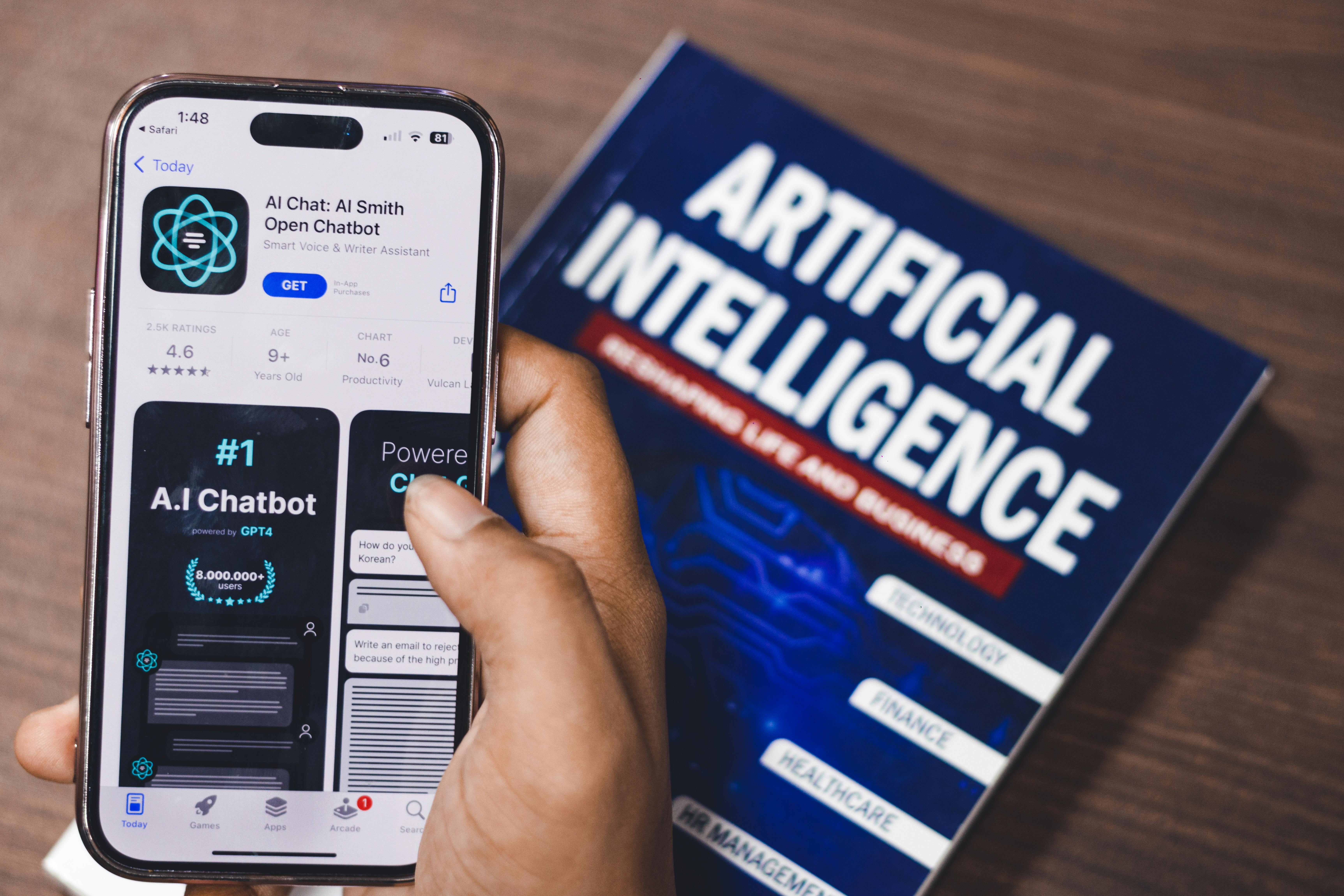
Fairness and Inclusivity in AI
Fairness in AI ensures that systems operate without bias or discrimination, especially against historically marginalized groups. The pursuit of fairness is both a technical and ethical challenge. Technically, developers must address issues such as biased datasets, unfair model assumptions, and unequal outcomes. Ethically, it requires engaging with questions of justice, representation, and human dignity. Bias in AI systems often originates from the data they are trained on. If the training data reflects existing social inequalities, these can be encoded and amplified in AI predictions. For instance, a facial recognition system trained predominantly on lighter skinned individuals may have significantly lower accuracy for darker-skinned faces. This leads not only to unequal service quality but also to serious risks, such as wrongful identification in law enforcement contexts.
Ensuring fairness means applying fairness metrics, statistical techniques to detect disparities in AI outputs across different demographic groups. These include demographic parity, equal opportunity, and individual fairness, each addressing fairness from different angles. But fairness also demands contextual awareness, understanding the specific social and historical dynamics of the domain in which the AI operates.
Inclusion, on the other hand, relates to the participation of diverse voices in AI development and governance. This includes gender diversity, racial representation, neurodiversity, and socio-economic diversity among development teams. Inclusive design ensures that AI systems are sensitive to the needs of all users, not just the dominant group. It also helps uncover hidden assumptions that may otherwise go unchallenged. A best practice in this regard is conducting algorithmic impact assessments, systematic evaluations of how an AI system might affect different stakeholders. This process, which mirrors environmental impact assessments, includes consultations with community groups, subject matter experts, and ethical review boards.
Privacy, Security, and Data Governance
AI systems thrive on data, but with data comes the critical responsibility of privacy protection and ethical data governance. In our increasingly digitized world, where data is collected through everything from smart devices to social media, ensuring privacy is a foundational ethical imperative.
Privacy is not merely a legal issue, it is a human right. AI systems must be designed to protect user autonomy, preserve anonymity when required, and prevent data misuse. This involves implementing strong data minimization policies (only collecting what is necessary), secure storage, and user control over how data is shared or used.
Security complements privacy. It refers to protecting AI systems from unauthorized access, adversarial attacks, and breaches that could compromise sensitive data or system behavior. A healthcare AI misdiagnosing a patient due to a cyberattack is not just a technical failure, it’s an ethical catastrophe. Data governance structures are needed to manage how data is sourced, labeled, stored, and accessed. Responsible data governance includes setting standards for transparency (e.g., data lineage and provenance), accountability (e.g., who is responsible for data breaches), and fairness (e.g., removing systemic bias from datasets).
One increasingly adopted framework is differential privacy, which enables AI systems to analyze data trends without exposing individual records. Another is federated learning, where models are trained across decentralized devices without transferring raw data to a central server, thereby preserving privacy. In high stakes domains, such as finance or justice, data used in AI systems must be auditable. Organizations should maintain documentation (often called “model cards” and “data sheets”) that describe the origin, structure, and limitations of the data and models they use. This transparency helps identify risks and improves public confidence in AI systems.
Transparency and Explainability
Transparency and explainability are essential for ensuring that AI systems are understandable and trustworthy. In many AI applications, especially those using deep learning, decisions are made through processes that are not easily interpretable by humans. This opacity creates what’s known as a "black box," where the inner workings of the system are hidden from users, developers, and regulators. Explainability means providing clear, meaningful information about how AI decisions are made. This is particularly important in high stakes situations, such as healthcare diagnoses, loan approvals, or parole decisions. When people are denied services or opportunities by an algorithm, they deserve an explanation they can comprehend and act upon.
Methods for achieving explainability include:
· Feature importance analysis, which shows which data inputs were most influential in a decision.
· Local Interpretable Model-agnostic Explanations (LIME), which approximates complex models with simpler ones for specific predictions.
· Counterfactual explanations, which answer the question: “What would have changed the outcome?”
· Model visualizations, including decision trees, heatmaps, and other graphical tools to demystify AI operations.
Beyond the technical layer, transparency also includes disclosure: Users should know when they are interacting with an AI system and how their data is being used. Organizations should also provide information on their AI models' capabilities, limitations, and potential risks.
From a governance perspective, transparency is a prerequisite for auditability. Regulators and oversight bodies need access to documentation and model behavior to evaluate compliance with legal and ethical standards. Increasingly, companies are being asked to publish transparency reports, detailing how their AI systems function and what steps they’ve taken to ensure fairness and accountability. Transparency builds public trust. In a world where misinformation and opaque technologies proliferate, offering clarity is not only ethical, it’s strategic.
Accountability and Contestability
Accountability ensures that someone can be held responsible when AI systems cause harm. In complex systems with many actors, from developers and data scientists to executives and policymakers, it’s easy for responsibility to become diluted. Ethical AI requires establishing clear lines of responsibility across the AI lifecycle.
There are several forms of accountability:
· Legal accountability, where laws define liability for harm caused by AI systems.
· Moral accountability, where organizations take responsibility for the societal impact of their technologies.
· Organizational accountability, where specific roles (e.g., AI ethics officer) are assigned to oversee responsible development.
Contestability means giving users the power to challenge or appeal AI decisions. This is especially important when AI systems make consequential decisions that affect people’s lives, such as eligibility for government benefits, job hiring, or immigration status.
To enable contestability, systems must:
· Keep audit trails records of how decisions were made.
· Offer appeals processes that involve human oversight.
· Provide access to inputs and reasoning used in the AI decision.
· Ensure timely resolution and responsiveness to challenges.
Building contestability into AI systems also improves their robustness. When feedback loops exist, organizations can learn from errors and refine their models to prevent recurrence. Without accountability and contestability, AI systems risk becoming instruments of unassailable authority, eroding democratic values and human rights. Ethical AI restores balance by ensuring systems remain subordinate to human judgment.
Regulatory and Ethical Frameworks
Effective governance of AI requires a combination of regulatory frameworks, ethical guidelines, and industry standards. Globally, governments and organizations are racing to develop policies that balance innovation with responsibility.
Some prominent frameworks include:
· Australia’s AI Ethics Principles, which offer voluntary guidelines for organizations to promote responsible AI development.
· EU’s Artificial Intelligence Act, which categorizes AI systems by risk level and applies proportionate regulatory requirements.
· OECD AI Principles, which promote inclusive growth, human-centered values, transparency, robustness, and accountability.
· IEEE Ethically Aligned Design, which provides recommendations for aligning AI with ethical principles across sectors.
These frameworks vary in scope and enforcement but share a common goal: to ensure that AI systems are aligned with fundamental rights and democratic values. Many of them emphasize the need for risk-based regulation, where the level of oversight depends on the potential harm of the AI application.
However, regulation alone is not enough. Ethical frameworks provide the moral compass that guides organizations beyond what’s legally required. These frameworks encourage self assessment, peer accountability, and public transparency. Leading companies are creating internal ethics boards, publishing AI principles, and training employees in ethical decision making. Ultimately, regulation and ethics must work hand in hand. Ethical principles guide behavior, while regulation enforces boundaries. Together, they create an ecosystem where responsible innovation can thrive.
Building a Culture of Responsible Innovation
Creating transparent and ethically responsible AI is not just a technical challenge, it’s a cultural transformation. Organizations must cultivate values that prioritize ethics, social impact, and human dignity alongside profitability and performance.
A culture of responsible innovation is one where ethical reflection is embedded into every stage of the AI lifecycle, from problem definition to design, deployment, and monitoring. This requires leadership commitment, interdisciplinary collaboration, and continuous education.
Steps to building this culture include:
· Establishing ethics boards or review committees that guide AI development.
· Hiring ethicists, social scientists, and community representatives into AI teams.
· Training staff on responsible AI principles and real world case studies.
· Incorporating ethical design patterns, such as user consent flows, fail safes, and equitable defaults.
· Providing feedback loops, where users can report issues or offer suggestions.
This cultural shift also means embracing humility and learning from failure. Organizations must be willing to revise or even withdraw AI systems that fail to meet ethical standards. Transparency in admitting mistakes and sharing lessons learned can enhance trust and inspire industry-wide improvements.
Finally, responsible innovation is about purpose. Why build this system? Whose life will it improve? By keeping humanity at the heart of technological progress, we ensure that AI serves as a force for good amplifying our values, supporting our communities, and creating a future that is not only intelligent but wise.
Your Next Step Starts Here
Got a bold idea or a tricky problem? We’re here to help. We work with individuals, startups, and businesses to design solutions that matter. Let’s team up and build something great together.
Your Next Step Starts Here
Got a bold idea or a tricky problem? We’re here to help. We work with individuals, startups, and businesses to design solutions that matter. Let’s team up and build something great together.
Your Next Step Starts Here
Got a bold idea or a tricky problem? We’re here to help. We work with individuals, startups, and businesses to design solutions that matter. Let’s team up and build something great together.